To keep up with the growing global demand for access to high-performance AI systems, the AI data center industry must continually integrate more powerful, more efficient hardware with tailored optical fiber networks designed to support AI’s complex workloads.
AFL’s latest white paper, AI Data Centers: Scaling Up and Scaling Out, provides an in-depth exploration of the technical building blocks enabling efficient scaling strategies in advanced AI data centers. This blog aims to introduce readers to the key themes discussed in the white paper, while highlighting the importance of industry collaboration across factors such as AI hardware innovation and modular infrastructure planning.
Understanding AI and Machine Learning
AI refers to machines designed to simulate human intelligence. These machines perform tasks that typically require human interaction (e.g., inferring sentiment, recognizing images, etc.). Machine Learning (ML) is a subset of AI split into two distinct phases: training and inference. These phases aim to develop algorithms capable of making predictions based on existing data. Large Language Models (LLMs) are AI systems that leverage Deep Learning (DL) to understand and generate human language (DL uses multi-layer neural networks to process and understand complex patterns in data).
Evolution of AI Since 2017
2017 marks a pivotal year in the establishment and rapid adoption of modern AI technologies. In that year, Google released a landmark paper, "Attention Is All You Need," introducing the Transformer model and ushering in a new era for natural language processing. The model uses self-attention to understand relationships and context over long distances in text (i.e., the model can understand and relate separated words or phrases throughout a given piece of text).
The Transformer’s high-performance architecture processes data in parallel (i.e., the model can manage multiple tasks simultaneously rather than completing tasks sequentially). This advanced capability significantly enhances both training times and scalability, establishing parallel processing as a cornerstone for various AI applications (e.g., text translation and text generation)
Understanding Scale Up and Scale Out
The challenges of expanding and enhancing advanced AI data center facilities to meet the immense computational demands of high-performance AI tasks underscore the critical importance of modular design.
Scaling up strategies focus on boosting the capacity of existing resources. This can involve adding more memory and upgrading servers with more efficient and powerful processors. Scaling out strategies aim to increase the available hardware by adding more data center servers (or nodes), thereby enhancing workload distribution and reducing redundancy. Together, effective scale-up and scale-out strategies form a comprehensive approach to achieving efficient, scalable growth in AI data centers.
Advances in AI Hardware
Significant innovations in AI hardware include:
- Semiconductor technology has seen remarkable progress, pushing the boundaries of semiconductor performance with new materials and fabrication methods enabling faster and more efficient AI computations.
- Chiplets offer an advanced and modular approach to chip design. With smaller, specialized chips that combine into complete systems, chiplets allow greater integration of technologies for enhanced flexibility and improved overall performance.
- Packaging techniques (i.e., methods to assemble and connect multiple components into single cohesive units) have also evolved significantly. By leveraging strategies such as stacking chips vertically and using high-speed interconnects, advanced packaging methods increase component density to enhance performance.
Future Trends in AI
Emerging trends in AI data centers include segmented models, less frequent synchronization, and extended distributed systems. The growing need for medium and long-haul links in Data Center Interconnect underscores the reliance of next-generation AI applications on robust networking solutions. Collectively, these trends signal an industry-wide shift towards more efficient and scalable AI infrastructures.
By addressing key themes such as hardware innovation and modular infrastructure planning, AFL’s white paper provides valuable, expert insights on scaling-up and scaling-out in the AI data centers. As the AI landscape continues to evolve, increased industry collaboration will become essential in meeting the complex, highly specialized demands of tomorrow’s AI data centers.
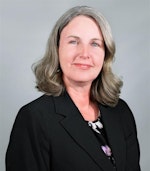
Manja Thessin
Manja Thessin RCDD/RTPM serves as Enterprise Market Manager for AFL, leading strategic planning and market analysis initiatives. Manja has more than 20 years of ICT experience in the field, design-and-engineering, and project management. She has managed complex initiatives in Data Center, Education, Industrial/Manufacturing and Healthcare. Manja earned a master’s certificate from Michigan State University in Strategic Leadership and holds RCDD and RTPM certifications from BICSI.
Guided by customer-first thinking, AFL has experienced remarkable growth coupled with outstanding market recognition. As a major player in the optical fiber hyperscale space, AFL contributes consistent industry messaging, offering readers the opportunity to stay current with emerging hyperscale technologies and the evolution of the AI data center landscape. AFL’s latest white paper, titled Advanced Networks for Artificial Intelligence and Machine Learning Computing: Scaling Fiber Networks to Meet Tomorrow’s Data Center Demands, enhances reader perceptions of networking for complex AI workloads.