Edge computing is not a single technology. Today we begin “Facets of the Edge,” a Data Center Frontier series examining the many components of edge computing, and the infrastructure needed to support them.
NEW YORK – “I’m going to challenge many of your beliefs,” says Simon Crosby. And he means it.
Crosby is the Chief Technology Officer of Swim, a startup focused on creating lightweight applications for data-intensive edge computing. Crosby believes that many edge applications can be managed by distributed networks of small, cheap computers like the Raspberry Pi and NVIDIA Jetson.
“Edge is not a device or a place,” Crosby said at the recent O’Reilly AI Conference. “It’s about how we compute. Edge applications must have a different paradigm.”
Edge computing extends data processing and storage closer to the growing universe of devices and sensors at the edge of the network. As we’ve often noted here at DCF, edge computing is not a single technology, but a phrase that describes several layers of infrastructure, some of which are refinements of existing models. Edge infrastructure will evolve in tiers, with opportunities in regional data hubs, small cities at telecom towers and on devices. Think of it in terms of “edge, edgier and edgiest.”
Crosby is among those who believe edge applications will require new thinking, and efficient use of resources. He noted that there are more than 5 billion Arm processors shipped each quarter, primarily for mobile devices and embedded systems.
“How will all these devices get smart?” Crosby asked. “Are we going to train these on the cloud and push them to the edge? There’s too much data. The workloads that are being (advocated) by AWS, Microsoft and Google represent a fictional data flow.”
Devices Are Becoming More Powerful
Instead, Crosby envisions distributed applications that can manage streams of data in real time, and use lightweight devices to run analytics locally, rather than pushing data to a cloud platform and back again. The Swim platform is designed to optimize artificial intelligence for the edge, using models that run locally on Arm-powered devices. Swim creates a fabric that abstracts a location and creates a “digital twin” for each real-world entity.
These digital twins require as little as 2 MB of disk space and can easily fit on Jetson or Raspberry Pi devices. This allows Swim to be deployed on a series of small nodes that can collect, transmit and model data.
The ability to bring AI onto edge devices has always been a wildcard in projections about the type of infrastructure needed for data processing and storage for edge computing. Data center startups are focusing on the opportunity for networks of micro-data centers in modules, while others see a boom at regional data centers. Meanwhile, a wave of hardware startups are designing new chips with the potential to shift AI data-crunching onto smaller devices.
It’s a trend predicted by data center veteran Scott Noteboom, the CEO of AI startup LitBit and a veteran of data center teams at Apple and Yahoo.
“Everything becomes a data center, because it has to,” Noteboom told us in 2017. “The majority of data center calculations and analytics will take place on the devices themselves.”
Swim demonstrates the potential power of this approach. One of its early deployments is in a real-time streaming traffic management system for the city of Palo Alto in Silicon Valley, which analyzes up to 30,000 data points per second from traffic lights, vehicles and pedestrians. “With just over 100 connected traffic intersections, Palo Alto’s traffic infrastructure will generate more data points per second than all of Twitter on any given day,” Swim says in a case study.
The economics of edge computing will emphasize cost and efficiency, with both compute and power. Small differences in form factor, processing power and latency will add up in distributed systems.
Swim builds web agents that possess individual logic, manage their own data streams, and continuously communicate their state. These agents are deployed at each intersection using a $200 NVIDIA Jetson edge processor, which updates the model and can analyze traffic patterns. The data stays local and maintains low-latency connections to other components in the system. Crosby says Swim processes 4 terabytes daily on edge devices in Palo Alto, which could cost $5,000 a month in a cloud deployment.
Swim has teamed with TrafficWare to create a product for this streaming traffic analytics application.
The Economics of Edge
Swim offers a specific approach to a particular problem. Many edge applications may require something different.
Crosby has experience with transformational technologies as one of the founders of XenSource, which popularized the widely-used Xen hypervisor and was later acquired by Citrix. He says that Swim takes an alternative approach to most cloud apps. “The problem is the advantage of cloud, which is more or less made possible by REST and statelessness and database centricity,” said Crosby. “These are your enemies (at the edge).
“The real world is stateful,” he continued. “We have to decide on the fly, and the data is often of ephemeral value. We’re moving from this world of Big Data to being required to find insights and act now. This is a different architectural approach for processing data on the fly.”
Swim’s backers include Arm Holdings, and it’s focused on technologies using Arm processors. Last year Arm and VNVIDIA announced plans to integrate the open-source NVIDIA Deep Learning Accelerator (NVDLA) architecture into Arm’s Project Trillium platform for machine learning, which will make it make it simpler for IoT chip companies to use Arm to integrate AI into their designs.
“Accelerating AI at the edge is critical in enabling Arm’s vision of connecting a trillion IoT devices,” said Rene Haas, executive vice president, and president of the IP Group, at Arm.
That’s a lot of devices, and serves as a reminder that the economics of edge computing will emphasize cost and efficiency, with both compute and power. Small differences in form factor, processing power and latency will add up in distributed systems.
“Here’s the problem: if your solution fits on two Raspberry Pis, you can’t charge a million dollars for it,” said Crosby.
The many facets of edge computing, along with the lengthy period during which they will be deployed, creates challenges in estimating the size of the market for specific technologies. and when the demand will materialize for its use. This makes it riskier to make detailed projections about edge computing and how the market will evolve. The focus on cost and efficiency also means that not all business models for hardware in traditional data centers will apply.
As edge business begins to arrive at scale, we’re also likely to see new hardware arriving, particularly in the realm of inference for AI workloads.
“There’s just a stupendous amount of compute coming down the pike, and it will all be available at the edge,” said Crosby. “There’s new exciting things coming from hardware.”
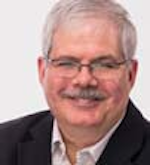