SANTA CLARA, Calif. – The leading hyperscale data center players continue to roll out new hardware designs to power machine learning applications. At the recent Open Compute Summit, Microsoft and NVIDIA unveiled a new hyperscale GPU accelerator for artificial intelligence workloads in the cloud.
The HGX-1 is an open source design that packs eight NVIDIA Tesla P100 GPUs in each chassis, with a switching design based on NVIDIA’s NVLink interconnect technology and the PCIe standard, which enables a CPU to dynamically connect to any number of GPUs (graphics processing units).
This approach allows cloud service providers using HGX-1 to offer customers a range of CPU and GPU machine instance configurations. Microsoft and NVIDIA built the new system in collaboration with Ingrasys, a subsidiary of Chinese hardware giant Foxconn.
The new hardware is part of Project Olympus, the Microsoft initiative to speed the development of open hardware optimized for cloud data centers, which features modular system binding blocks and early access to designs for the Open Compute Project community.
Microsoft Likes Extreme Scalability
“The HGX-1 AI accelerator provides extreme performance scalability to meet the demanding requirements of fast-growing machine learning workloads, and its unique design allows it to be easily adopted into existing data centers around the world,” wrote Kushagra Vaid, general manager and distinguished engineer, Azure Hardware Infrastructure, Microsoft, in a blog post.
The HGX-1 wasn’t the only AI hardware introduced at the Open Compute Summit. The Facebook infrastructure team unveiled its Big Basin machine learning server, which also features eight NVIDIA Tesla P100 accelerators connected by NVLink.
In artificial intelligence (AI), computers are assembled into neural networks that emulate the learning process of the human brain to solve new challenges. It’s a process that requires lots of computing horsepower, and hyperscale data center operators are using GPUs, FPGAs and ASICs offer more sophisticated processing architectures that can bring HPC-style parallel processing of workloads. This is especially important in machine learning, where neural networks must be trained by absorbing large datasets and refining its algorithms for accuracy. Once a system is trained, it uses inference to “learn” as it encounters new information.
NVIDIA Sees New Standard
NVIDIA’s graphics processing (GPU) technology has been one of the biggest beneficiaries of the rise of specialized computing, gaining traction with workloads in supercomputing, artificial intelligence (AI) and connected cars.
A CPU consists of a few cores optimized for sequential serial processing, while a GPU has a parallel architecture consisting of hundreds or even thousands of smaller cores designed for handling multiple tasks simultaneously. This has proven ideal for the data crunching required to train and power AI workloads.
A Microsoft image of the HGX-1 chassis design, configured with eight NVIDIA Tesla P100 GPUs using the NVLink interconnect, which is faster than PCIe. (Photo: Microsoft)
NVIDIA says the HGX-1 design creates a standard architecture for cloud-based AI computing, comparing its potential impact to ATX (Advanced Technology eXtended), which forged a standard for PC motherboards when it was introduced in the 1990s.
“AI is a new computing model that requires a new architecture,” said Jen-Hsun Huang, founder and chief executive officer of NVIDIA. “The HGX-1 hyperscale GPU accelerator will do for AI cloud computing what the ATX standard did to make PCs pervasive today. It will enable cloud-service providers to easily adopt NVIDIA GPUs to meet surging demand for AI computing.”
Huang says the modular chassis design of the HGX-1 provides flexibility to reorganize components to support diverse workloads. AI training, inferencing and HPC workloads run optimally on different system configurations, with a CPU attached to a varying number of GPUs.
Intel Targets FPGAs for Cloud, AI
Intel is also raising its game to remain competitive in the AI hardware sector, and was at the Open Compute Summit showing off a Project Olympus design combining traditional Intel CPUs with field programmable gate arrays (FPGAs), semiconductors that can be reprogrammed to perform specialized computing tasks. FPGAs allow users to tailor compute power to specific workloads or applications.
Intel’s Jason Waxman shows off a server using Intel’s FPGA accelerators with Microsoft’s Project Olympus server design during his presentation at the Open Compute Summit. (Photo: Rich Miller)
Intel gained a strong position in FPGAs with its $16 billion deal to acquire Altera in 2015. Microsoft is now using FPGAs to accelerate its cloud infrastructure using designs from Project Catapult. Interestingly, Microsoft has focused on harnessing FPGAs to speed its Bing and Azure operations rather than machine learning.
The November 2016 launch of the Intel Deep Learning Inference Accelerator (DLIA) marked Intel’s first product release using Altera FPGA tech. Intel will further broaden its offerings for the AI market later this year when it introduces its Nervana platform, backed by ASICs (Application Specific Integrated Circuits) that are highly tailored for machine learning. ASICs are customized for specific workloads, but cannot be reprogrammed, as is the case with FPGAs.
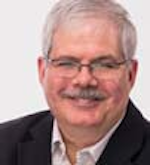