Intel Unveils FPGA to Accelerate Neural Networks
Intel today unveiled new hardware and software targeting the artificial intelligence (AI) market, which has emerged as a focus of investment for the largest data center operators. The chipmaker introduced an FPGA accelerator that offers more horsepower for companies developing new AI-powered services.
The Intel Deep Learning Inference Accelerator (DLIA) combines traditional Intel CPUs with field programmable gate arrays (FPGAs), semiconductors that can be reprogrammed to perform specialized computing tasks. FPGAs allow users to tailor compute power to specific workloads or applications.
The DLIA is the first hardware product emerging from Intel’s $16 billion acquisition of Altera last year. It was introduced at SC16 in Salt Lake City, Utah, the annual showcase for high performance computing hardware. Intel is also rolling out a beefier model of its flagship Xeon processor, and touting its Xeon Phi line of chips optimized for parallelized workloads.
“We’re really jazzed about the promise of FPGAs,” said Charles Wuischpard, Vice President, Scalable Data Center Solutions Group. “You can’t create a custom chip for every workload you come across. There’s a balance between customization and general purpose.”
Targeting AI Inference Workloads
Machine leaning involves two types of computing workloads with different profiles, known as training and inference. Both involve neural networks – groups of computers that mimic the way neurons work together in the human brain.
- In training, the network learns a new capability from existing data. Training is compute-intensive, requiring hardware that can process huge volumes of data.
- In inference, the network applies its capabilities to new data, using its training to identify patterns and perform tasks, usually much more quickly than humans could.
The Intel DLIA hardware and software is designed to accelerate inference workloads using convolutional neural networks, which are used for image recognition (like when Facebook identifies your friends in a photo you’ve just uploaded). Intel says the FPGA hardware, which will be available in 2017, will offer “exceptional throughput and power efficiency.”
Intel sees FPGAs as the key to designing a new generation of products to address emerging customer workloads in the data center sector, especially in artificial intelligence, an area where rival NVIDIA has gained strong traction.
Why FPGAs Matter to Intel
FPGAs can serve as coprocessors to accelerate CPU workloads, an approach that is used in supercomputing and HPC, usually by teaming CPUs with NVIDIA GPUs or Intel’s x86-based Xeon Phi coprocessors. Altera was also a leading player in programmable logic devices (PLDs), which are widely used to automate industrial infrastructure.
The DLIA is a PCiE card featuring the Arria 10 FPGA developed by Altera. Its architecture enables remote updates so the hardware can keep up with the rapid pace of innovation in AI.
“The FPGA is a great product for this market, but it does take a high level of programming capability,” said Barry Davis, General Manager of Intel’s Accelerated Workload Group. “Algorithms are changing all the time. This allows us to provide software updates to our users.”
“AI really is a new wave of computing,” said Davis. “That wave has arrived. With the improvements in hardware and the vast amounts of data, there’s a surge in AI as a capability for analytics and public cloud processing.”
On the customer front, a particular focus is the “Super 7” group of cloud service providers that are driving hyperscale infrastructure innovation. This group includes Amazon, Facebook, Google and Microsoft, along with Chinese hyperscale companies Alibaba, Baidu and Tencent.
Intel’s Diane Bryant has said that four of the seven companies are expected to sample the new CPU/FPGA products.
The integration of FPGAs is a key strategy for Intel as it sharpens its focus on chips for these large data centers. Intel projects that 70 to 80 percent of systems will be deployed in large-scale data centers by 2025.
Hot Competition in AI Hardware
The appetite for accelerated computing for AI was clearly visible last week in the earnings of NVIDIA, which reported a 193 percent increase in its revenue from data center customers. NVIDIA’s GPUs are now used in cloud services from Amazon, IBM, Microsoft and Alibaba, as well as the massive machine learning operation at Facebook.
Wuischpard says competition is nothing new for Intel, noting the philosophy of the late Andy Grove, Intel’s former CEO, who wrote a book titled “Only the Paranoid Survive.”
“We see competition as being a good thing,” said Wuischpard.
That’s why Intel is moving to acquire new technology to reshape its roadmap. Altera was the first major move in this effort, and was followed by Intel’s purchase of Nervana, a startup specializing in specialized computing for AI workloads.
Nervana provides expertise with ASICs (Application Specific Integrated Circuits) that are highly tailored for machine learning. ASICs are customized for specific workloads, but cannot be reprogrammed, as is the case with FPGAs.
Intel’s Naveen Rao, the CEO and co-founder of Nervana, speaks at the O’Reilly AI conference in New York. (Photo: Rich Miller)
Nervana is also developing an ASIC that it says has achieved training speeds 10 times faster than conventional GPU-based systems and frameworks. The company says it has also built a better interconnect, allowing its Nervana Engine to move data between compute nodes more efficiently.
“The constraints are actually what we can do with the existing hardware,” said Naveen Rao, founder of Nervana, during a talk last month at the O’Reilly AI conference in New York. “One of the fundamental problems is we want to scale over multiple processors.”
The first Nervana-based Intel hardware project is expected in 2017. In the meantime, Intel has released a new Xeon processor SKU for SC16. The Xeon E5 2699A is an enhanced Bradwell processor positioned at the top end of Intel’s Xeon product tier.
It’s All About the Roadmap
“We’re taking a full solution approach to this,” said Davis. “It’s not just about component technology or parts of software. It is a complex space, but we’re building on our experience in HPC. All of our products are brought to bear here.”
Amitai Armon, Intel’s Chief Data Scientist for Advanced Analytics, discussed the company’s approach to AI hardware at the O’Reilly AI conference.
“We need to understand the needs of this domain, which is not an easy task,” said Armon. “In four or five years, the most popular algorithms will probably be different.
“The compute building blocks for machine learning have remained the same over time,” he said. “Compute is not the whole story. The hardware bottleneck is often not the compute, but the memory bandwidth.”[clickToTweet tweet=”Intel’s Amitai Armon: Compute is not the whole story. The hardware bottleneck is often the memory bandwidth.” quote=”Intel’s Amitai Armon: Compute is not the whole story. The hardware bottleneck is often the memory bandwidth.”]
That’s why the Xeon Phi product for HPC features more memory bandwidth on die, about twice that of Xeon e5 v4. This makes it well suited for AI training, in which algorithms absorb huge amounts of data
The latest Xeon Phi processor was selected for nine new systems on the supercomputing Top500, including two systems in the Top 10 (the fifth place Cori system, and an Oakforest-PAC system ranking sixth).
Intel will further boost its machine learning chops with the next version of Xeon Phi, known as “Knights Mill,” which will arrive next year and be optimized for deep learning, The Nervana hardware will also boost memory management.
This reflects Intel’s emphasis on flexible solutions that address a broad array of workloads over a lengthy period of time.
“You don’t get into this game for just one processor,” said Wuischpard. “It has to be a roadmap. Our pipeline of projects stretches for more than a decade.”
About the Author
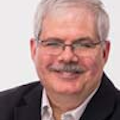