Artificial intelligence is one of the hottest technology trends for 2017. And perhaps no company in the AI sector is hotter than NVIDIA, which has pushed from the desktop into the data center, evolving into a major player in high performance computing.
NVIDIA’s graphics processing (GPU) technology has been one of the biggest beneficiaries of the rise of specialized computing, gaining traction with workloads in supercomputing, artificial intelligence (AI) and connected cars. This trend is expected to accelerate in 2017, with more custom chips being introduced to target these workloads.
After building a major beachhead in hyperscale data centers, NVIDIA’s ambitions now extend to the enterprise data center. The company’s new DGX-1 Deep Learning System is a “supercomputer in a box” – a hardware appliance designed to make AI data crunching more accessible.
The DGX-1 is a 3U appliance packed with 8 Tesla P100 GPUs, each with 16 GB of memory, providing 170 teraflops of computing power in a footprint of 3.2kW of power. It’s an integrated solution that includes hardware, deep learning software, development tools, and AI-accelerated analytics applications. NVIDIA believes the DGX-1’s combination of compute power and energy efficiency can democratize AI computing hardware, most of which currently runs in research labs or the hyperscale data centers of cloud providers, who deliver machine learning as a service.
“AI has begun with the big cloud service providers, but I don’t think it’s far-fetched that enterprises will work with AI,” said Jim McHugh, VP and General Manager at NVIDIA. “In the next three years, 20 percent of enterprises will have people working on neural networks. The other 80 percent are in trouble.”
Big Investments in Specialized Computing
In artificial intelligence (AI), computers are assembled into neural networks that emulate the learning process of the human brain to solve new challenges. It’s a process that requires lots of computing horsepower, which is why the leading players in the field have moved beyond traditional CPU-driven servers.
The race to leverage AI is led by the industry’s marquee names – including Google, Facebook, Amazon and Microsoft – who are seeking to add intelligence to a wide range of services and applications.
As usual, the battlefield runs through the data center, with implications for the major cloud platforms and chipmakers. Some like Google, are designing their own silicon and hardware for AI workloads. Others, like Facebook and Microsoft, are working closely with either NVIDIA or Intel on new server designs to leverage specialty chips.
Facebook’s Big Sur AI server is a 4U chassis packed with up to eight NVIDIA M40 GPUs (Photo: Rich Miller)
NVIDIA has been investing heavily in innovation in AI, which it sees as a pervasive technology trend that will bring its GPU technology into every area of the economy and society.
“There are so many use cases,” says McHugh. “People are using AI every day. AI is for everyone.”
From the Desktop to the Data Center
When you mention NVIDIA, many people think of PC graphics cards. The company was founded in 1993, and its graphics processing units (GPUs) quickly became an essential tool for gamers yearning for more horsepower. The company’s GPUs worked with CPUs, but took a slightly different approach to processing data. A CPU consists of a few cores optimized for sequential serial processing, while a GPU has a parallel architecture consisting of hundreds or even thousands of smaller cores designed for handling multiple tasks simultaneously.
Around 2009, GPU acceleration was adopted in high performance computing (HPC), enabling server clusters and supercomputers to perform more parallel processing. In 2010, NVIDIA’s Tesla GPUs accelerated the world’s most powerful supercomputer, China’s Tianhe-1.
NVIDIA’s Jim McHugh sees many developing technologies that will boost demand for HPC in edge environments. (Photo: Rich Miller)
Parallel processing also turned out to be important in the burgeoning field of artificial intelligence, helping NVIDIA quickly gain a foothold. Facebook picked NVIDIA GPUs to power Big Sur, its custom hardware for AI. Facebook can train its machine learning systems to recognize speech, understand the content of video and images, and translate content from one language to another.
The leading cloud players are offering interest in GPUs, as Amazon Web Services, Microsoft Azure, Google Cloud Platform and IBM all offer GPU cloud servers. The cloud-powered “as a service” model has become an entry point for many companies seeking to leverage AI and machine learning, since few have the in-house capability to run specialized hardware for AI workloads.
The appetite for accelerated computing was clearly visible in NVIDIA’s latest quarterly earnings, which showed a 193 percent increase in its revenue from data center customers.
Intel, IBM Step Up Their Game
The market for specialized computing is getting more competitive. Intel recently introduced an FPGA accelerator that combines traditional Intel CPUs with field programmable gate arrays (FPGAs), semiconductors that can be reprogrammed to perform specialized computing tasks. FPGAs allow users to tailor compute power to specific workloads or applications.
Intel acquired Altera to beef up its FPGA capabilities, and then bought AI startup Nervana adds expertise with ASICs (Application Specific Integrated Circuits) that are highly tailored for machine learning.
IBM is also focused on AI and “cognitive computing,” championing its Watson supercomputing platform for delivery of cloud-driven Meanwhile, Google has developed the Tensor Processing Unit (TPU), a custom ASIC tailored for TensorFlow, an open source software library for machine learning that was developed by Google.
NVIDIA’s McHugh sees opportunities for disruption in many areas of the economy, including the data center sector.
“We’re entering a new generation of compute and a new generation of AI,” said McHugh. “We’re hitting a point where AI is changing things in many industries.”
Why AI Workloads Are Different
Artificial intelligence involves two types of computing workloads with different profiles, known as training and inference. Both involve neural networks – groups of computers that mimic the way neurons work together in the human brain.
- In training, the network learns a new capability from existing data. Training is compute-intensive, requiring hardware that can process huge volumes of data.
- In inference, the network applies its capabilities to new data, using its training to identify patterns and perform tasks, usually much more quickly than humans could.
The NVIDIA DGX-1 on display at the Gartner Data Center conference in Las Vegas. (Photo: Rich Miller)
The two tasks require different types of hardware: data-crunching horsepower for training, and latency and performance for inference. This is significant because it guides how these systems are deployed, and where they reside.
NVIDIA’s positioning of DGX-1 as entry point in the enterprise data center is an intriguing development for the data center industry.
“With DGX-1, we’ve taken learning from the largest cloud service providers and are providing it to enterprises in the form of a software and hardware stack that can be optimized,” said McHugh.
To demonstrate the new system, NVIDIA created the DGX SATURN V, bringing together 125 DGX-1 systems to create a supercomputer. The SATURN V system was named the most efficient supercomputer in the world in the annual Green 500 rankings, and is No. 28 on the Top500 power rankings.
NVIDIA has delivered DGX-1 systems to Massachusetts General Hospital, artificial intelligence labs at Stanford and Cal-Berkeley and the Open AI Institute, where NVIDIA CEO Jen-Hsun Huang personally delivered the first production model to Open AI sponsor Elon Musk.
On the Horizon: The IoT and Autonomous Vehicles
As artificial intelligence is applied to create smarter devices and services, it will cross over into the Internet of Things.
“The world of the Internet of Things is going to be changing drastically with AI because it allows us to train applications at the edge, so they are intelligent,” said HcHugh. “The volume of data we’re dealing with the world of IoT can’t be processed manually. It has to be automated, and AI will play a large role in this.”
That’s why NVIDIA sees the IoT as a potential bridge between the “AI as a Service” cloud services and corporate and service provider data centers.
“As people develop large datasets and don’t want to move them, they will start to train the dataset on premises, and then do inference in the cloud,” said McHugh. “A big part of training is data locality.”
Perhaps the most promising market for NVIDIA is connected cars and autonomous vehicles. Tesla, Uber, GM, Apple and Mercedes are among the companies developing autonomous vehicles, which could create radical change in American transportation and urban life. There are huge challenges and resistance points, but technologists say autonomous cars offer an enormous societal benefit. If self-driving vehicles can be even slightly safer than humans, it could translate into saved lives.
“Autonomous driving will usher in a world that is safer,” said McHugh.
The the ZF ProAI self-driving system, which is based on the NVIDIA DRIVE PX 2 AI computing platform, on display at the recent Detroit Auto Show. It will be the first tier 1 production system for companies building self-driving vehicles. (Photo: NVIDIA)
NVIDIA has been developing its own self-driving vehicle, known as BB8, in its research facility in Holmdel, N.J. It also is working with numerous automakers, including Audi, Tesla, Volvo, Mercedes Benz, Baidu, Honda, BMW. It also is partnering with tech companies targeting the autonomous driving market, including nuTonomy and WEpods.
“We think of autonomous cars, but the transportation market is much larger than just cars,” said McHugh. “Trucks and shuttles will be one of the earliest implementations. The market is huge. Transportation is a $10 trillion industry. Trucking and shuttles is the largest part of it.”
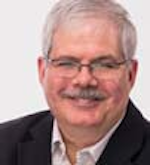