For Leading Cloud Platforms, AI Presents a Major Opportunity
Getting AI into your business can be expensive. It requires being able to make the most of your data resources, understanding how AI can improve your workflows and the benefits it will bring to business and customer. Hardware resources for AI are expensive, especially the specialty chip technologies that provide the greatest bang for the buck. Almost no one is going to jump headfirst into developing the infrastructure necessary to support an integrated AI workflow approach.
And that's where the big cloud providers see an opportunity. They can deliver the storage necessary for companies to use their own data to optimize their AI workflow, they have the infrastructure and the methodology to deliver capacity on-demand to their customers, and they've been building AI workflows for their own businesses and are now in a position to sell the knowledge and techniques they've learned to their customers.
While there are a growing number of companies offering you AI resources, from hardware clouds to software stacks (and we will cover them in our next story), most of us are probably dealing with the big three when we look at AI and the cloud: AWS, Google, and Microsoft. Nvidia may be the dominant player in the AI hardware business, but when it comes to cloud services they are just trying to break into the market.
Whether you like it or not, you are probably interacting with AI every day in your business life. Be it intentionally, because you choose to use ChatGPT to search, answer questions, or do a bit of coding, or unintentionally, when you click on a link or product recommended by the inference engine utilized by a site you frequent, AI is slipping into our daily lives.
Amazon Web Services
AWS offers more than 20 pre-trained AI services that can provide intelligence for your applications and services without the need for your business to train up new models. These tools do not require specific machine learning experience and benefit from being run on the same technology as Amazon.com and their ML services, which provides an ongoing learning environment for the services you choose to use without the customer needing to contract for or work on additional learning tasks.
The services cover computer vision, automated data extraction and analysis, language AI, customer experience, business metrics, code and DevOps, and industrial AI. By utilizing AWS for these services businesses can build and deploy sophisticated AI models and applications without the need for extensive AI expertise or its very expensive infrastructure.
Customers can use any or all of the specific services offered by Amazon to meet their business needs. The lead development service is Amazon Sagemaker, which is a fully-managed platform for building, training, and deploying machine learning models at scale. There's also a large selection of partners who offer consulting and development services for customers looking to use the AWS AI Service, such as Torch.AI, Cognizant, Wipro, and Trifacta.
AWS also works with entities like Hugging Face AI community to offer the ability to use the Hugging Face Transformer architecture models with Amazon Sagemaker on the AWS platform to speed up deployment of NLP models. Other partners have focused on offering more specific capabilities such as focusing on security analytics or data mining.
The biggest advantage for AWS is that they offer their services not just for ML experts or data scientists, but have focused on tool interface and usability to enable business users to find ways to integrate the AI services into their business workflows.
Google offers more than 20 AI products, services, and solution sets via Google Cloud, with a primary focus on developers and data scientists. Most people are likely to be familiar with Google Bard, their browser-based conversational AI that was pushed to market in response to the release of ChatGPT and Microsoft’s heavy investment in that technology. Bard is still considered experimental and is not a full-fledged service available to customers at this point in time.
Google defines their available services by use case, breaking those down by data scientist, developer, infrastructure, business solutions, and consulting. Infrastructure is where customers get access to just that; preconfigured deep learning containers and deep learning VMs to run on the Google cloud, but also access to high performance GPUs, Google’s in-house developed hardware accelerated TPUs, and TensorFlow enterprise, which allows users to scale resources across CPUs, GPUs, and TPUs.
This infrastructure supports Googles AI tools, from their unified ML development platform, Vertex AI, to their AutoML tool for training custom ML models. As you would expect developers have access to NLP, translation, video and vision tools to utilize in their application development and deployment.
While Googles focus has been on developers and data scientists, they do offer a small selection of packaged cloud AI solutions targeted at the business user. Their Contact Center AI, Documents AI, and Product Discovery solutions contain pre-trained solutions for common tasks that businesses would use AI for in these situations and give businesses a starting point for the integration and deployment of Google AI solutions.
Their consulting services can build on any of the existing Google solution products as well as offering their AI readiness Program, a customized AI “boot camp” to help jump start your business into the AI world that works with your team to give you the tools necessary to start your AI development journey.
Microsoft
Microsoft Azure AI is a collection of AI services for developers and data scientists. Like AWS and Google, training, NLP, vision, and speech services are available through supported API calls. Users can create their own ML models to run on their selected level of AI supercomputer infrastructure. Commonly used frameworks such as PyTorch and TensorFlow are supported across the Azure AI infrastructure.
Azure AI is broken into four service areas; Applied AI Services, Cognitive Services, Machine Learning, and Infrastructure. Applied AI services are focused on task-specific AI designed to solve scenarios for common business activities. Cognitive Services are focused on vision, speech, language and decision making. This is where the well-known OpenAI models fit in, as one of the cutting-edge AI models available for customer use. Machine learning is the end-to-end platform for the development, training and deployment of ML models and allows the creation and deployment of these models at scale.
Infrastructure is a purpose-built supercomputing infrastructure that offers a range of acceleration hardware, OUs, and Tensor cores, ranging from what are effectively entry-level AI supercomputing on up to Nvidia A100 clusters, with plans to introduce newer Nvidia H100 DGX clusters later this year. Customers can select from a range of AI-optimized VMs making use of the hardware infrastructure making use of, and paying for, the level of supercomputing power that they need to meet their business goals and timeframes.
Like the offerings from Amazon, the available tools and solutions are also used within the company’s own environment, providing the AI backend for customer facing products including those found in the Microsoft 365 desktop applications.
…and the rest
While it may seem strange to see these vendors at the bottom of the story, this isn’t a comment on capabilities as much as it is perception of the customers.
Oracle Cloud Infrastructure AI Services
The Oracle Cloud Infrastructure AI services are best known for their chatbots, prebuilt conversational digital assistants that do a good job of working with natural language processing. OCI offers the full range of AI development and deployment services and is the launch platform for the Nvidia DGX cluster, the purpose-built H100 based AI supercomputer.
IBM Watson Studio
Prior to the most recent round of AI furor if you asked people what they thought of when AI computers were brought up there would be an excellent chance they would mention IBM’s Watson playing Jeopardy or Deep Blue playing chess. Well, the IBM Watson studio is the descendent of those earlier AI-like iterations and offers customers the opportunity to create their own AI solutions with similar features to their competitors.
Their big difference is a reflection of IBMs focus on hybrid computing. Watson Studio runs on the IBM Cloud Pak for Data, which is a cloud-native platform, available as both as-a-Service and an on-premises configuration, so that customers can have their own on-site deployment of the technology should there be technology or security reasons that this makes the most sense.
Special purpose clouds
There are a number of AI clouds that offer services to specific industries or technologies. Some examples of this include Outmatch, which uses AI to improve the employee recruitment process, Symantec TAA Targeted Attack Analytics, which uses cloud-based AI to support it advanced threat protection, H2O which offers an AI cloud that looks at open source solutions for corporate use, and Salesforce Einstein, a generative AI solution specifically for CRM.
Alibaba Cloud, Baidu AI Cloud, Tencent Cloud Platform
These three Chinese companies, all of whom built their name in areas other than AI, are focusing significant resources in the development and deployment of competitive cloud AI services.
There are no less than two dozen additional companies fighting for their share of the AI cloud space, both as software and hardware solutions. For now, cloud solutions offer the most cost-effective way for business to begin to evaluate, test, and deploy AI tools and solutions while focusing on gaining that competitive business advantage.
About the Author
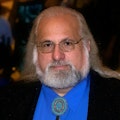