The AI Hardware Startups Are Coming. Intel Plans to be Ready
NEW YORK – The market for artificial intelligence hardware is entering a new phase, with incumbents competing hard for every workload, even as a cluster of startups prepare to bring specialized AI chips to market.
“At the end of this year, we expect to see a number of companies introduce new hardware for inference with lower energy use,” said Roger Chen, CEO of Computable Labs, said at the kickoff of last week’s O’Reilly Artificial Intelligence Conference, where the new hardware for distributed AI was a hot topic.
“There’s just a stupendous amount of compute coming down the pipe, and it will all be available at the edge,” said Simon Crosby, the Chief Technology Officer at SWIM, which designs software for intelligent edge applications.
The arrival of startup silicon on the AI computing market follows several years of intense competition between chip market leader Intel Corp. and rivals including NVIDIA, AMD and several players advancing ARM technology. Intel continues to hold a dominant position in the enterprise computing space, but the development of powerful new hardware optimized for specific workloads has been a major trend in the high performance computing (HPC) sector, boosted by demand for data-crunching for artificial intelligence and other types of specialized workloads.
Beneficiaries include NVIDIA and AMD, as well as ARM specialist Ampere and a host of startups developing low-power chips to allow smartphones to run AI workloads on the device.
Intel also believes the market is evolving, but expresses confidence in its opportunities in AI, particularly as more compute is applied to inference (decision-making) in addition to training algorithms.
“We are at the start of a new phase,” said Gadi Singer, vice president of the Artificial Intelligence Products Group and general manager of architecture at Intel. “Deep learning, as a technology, is changing the value compute brings to individuals and organizations.
“AI hardware has been advanced,” said Singer. “The focus now goes to inference, and most of the inference in the data center runs on CPUs. This allows companies to use the hardware they have, and add new (accelerator capacity) as they look to enhance the hardware they have.”
The AI Boom Continues
In artificial intelligence (AI), computers are assembled into neural networks that emulate the learning process of the human brain to solve new challenges. It’s a process that requires lots of computing horsepower, which is why the leading players in the field have moved beyond traditional CPU-driven servers.
The race to leverage AI is led by the industry’s marquee names – including Google, Facebook, Amazon and Microsoft – who are seeking to add intelligence to a wide range of services and applications.
Venture capitalists and entrepreneurs are also focused on artificial intelligence. Close to 2,000 companies have raised $19 billion in equity to fund startups in AI-related software or services, according to Deepashri Varadharajan, the Lead Analyst for AI at CB Insights. She said one of the most important investment sectors is new hardware.
“Today at least 40 different startups have raised capital to develop AI hardware,” said Varadharajan. “Very narrow focus areas are beginning to emerge, and it is changing the competitive landscape. Five years ago Intel competed with other large manufacturers, like AMD and NVIDIA and ARM.”
The crop of AI hardware startups is spread across the spectrum, including many focusing on edge computing workloads. Here’s an overview of some of the most prominent startups, with links to media coverage:
- SambaNova Systems: This startup featuring technology from two Stanford researchers Kunle Olukotun and Chris Ré made headlines by raising more than $200 million in its Series A and B rounds, with backing from Intel and Google Ventures.
- Graphcore: This UK-based AI startup has raised more than $300 million in venture capital and is valued at $1.7 billion. Its investors include BMW and Microsoft, and its technology features a custom chip known as an Intelligence Processing Unit (IPU).
- Cerebras Systems: This startup, still in stealth mode, is headed by Andrew Feldman, who founded low-energy chip startup SeaMicro and sold it to AMD. Read more at Barron’s.
- Habana Labs: This fabless semiconductor startup is developing a purpose-built inference processor. The Next Platform has an overview.
- Wave Computing: This startup describes its chips as DPUs (DataFlow Processor Units) that can extend an AI platform from the edge to the data center. Here’s a summary from The Next Platform.
- Mythic: This Austin-based startup making hardware to power AI on devices, and does its processing in flash memory so it can put more power in a device without generating heat and draining the battery.
- Groq: This stealthy startup was founded by former Google engineers and is reported to be developing a tensor processing unit (TPU), the same class of chip running Google’s AI workloads. It has raised $52 million from Social Capital.
- FlexLogix: Backed by Lux Capital and Eclipse Ventures, this startup is using an FPGA to target accelerated inference for edge computing. See coverage from ZDNet
- Cornami: This Santa Clara startup is building chips for both training and inference using a systolic array. See coverage from ZDNet
- Gyrfalcon Technology: A Milpitas, Calif. startup developing energy-efficient silicon for edge inferencing. See more from ZDNet.
- Efinex: Another startup focused on edge inferencing for the Internet of Things, leveraging both FPGAs and ASICs. Coverage from ZDNet.
Matching Compute to Specialized Workloads
These AI startups could accelerate the ongoing shift towards specialized computing hardware, featuring custom chips like graphics processing units (GPUs), field programmable gate array (FPGAs) and application-specific integrated circuits (ASICs) in addition to the general purpose x86 CPUs that currently dominate the IT landscape.
This was one of the trends highlighted by researcher Suvojit Ghosh of McMaster University in Hamilton, Ontario, in a recent presentation at DCD New York.
“What we will probably see is a move to silicon that is optimized for workloads,” said Ghosh, who cited GPUs as being particularly cost-efficient in managing dense loads.
Many of these startups aspire to shift computing power and algorithms out of the data center and onto smartphones and tablets, as well as appliances on factory floors and hospitals. The evolution of edge devices and “fog computing” – processing power near the perimeter of the network – will play a role in the geography of the Internet of Things, helping to deliver capacity to billions of devices and sensors.
Some context on chips and AI workloads is helpful in handicapping how this market might evolve beyond traditional CPUs, where Intel has dominated. GPUs have a parallel architecture consisting of hundreds or even thousands of smaller cores designed for handling multiple tasks simultaneously. FPGAs and ASICs are semiconductors that can be customized to perform specialized computing tasks, allowing users to tailor compute power to specific workloads or applications.
There are several types of AI computing workloads. In training, the network learns a new capability from existing data. In inference, the system applies its capabilities to new data, using its training to identify patterns and perform tasks, usually much more quickly than humans could.
Intel’s Plans for a Changing Landscape
Intel’s message is simple: Our platform allows you to get the most mileage from the compute you already operate, while leaving room to hardware accelerators for targeted workloads.
Singer says Intel will introduce several models of its NNP neural processor accelerator later this year, with the NNP-I 1000 addressing inference, and NNP-L 1000 optimized for training. The NNP I-1000 is currently being used by Facebook and serving “tens of trillions of inference processing transactions,” said Singer.
Singer reinforced the conviction that edge computing is complementary to traditional data centers, and more powerful device-based compute power won’t alter that structure.
“Local data centers are not going to go away,” said Singer. “The growth is going to happen across the whole computing continuum. The scale of inference, along with the economics, argues for having that happen at the edge. There will be more deep learning happening at the edge of the network.
“There is also a strong case for aggregation, which will be seen in data centers,” he added. “We see all of those use cases growing as deep learning becomes a larger component of demand across all these venues. We plan to have products across these compute spaces.”
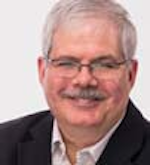