Our 2020 podcast season kicks off with a series of shows based on DCF’s annual forecast, which we call “Eight Trends That Will Shape the Data Center in 2020.”
On this podcast, we explore two of the most important trends: data tonnage, and the hardware arms race around artificial intelligence, or AI.
Our number one trend is that in 2020 the explosive growth of data will be felt like never before. Data tonnage creates challenges in both the distribution and concentration of data.
Also, artificial intelligence (AI) plays a starring role in this data tsunami. AI is a hardware-intensive computing technology that will analyze data both near and far. That includes everything from algorithm training at cloud campuses to inference engines running on smartphones.
Our podcast host, Rich Miller dives deeper into both these subjects which will definitely impact the data center.
A disk tray for a Facebook high-capacity storage server. (Photo: Rich Miller)
So let’s talk about data. It’s the reason data centers exist, and the driving force behind the growth of the industry. The reason we are always building data centers is that our world is being transformed by data – and this digital transformation is generating an ocean of data, fed by a growing universe of intelligent things – that includes sensors, cameras, your smartphones and tablets, and the early versions of drones, robots and connected cars.
Our number one trend is that in 2020 the explosive growth of data will be felt like never before. We believe this is a sign of things to come, as next-generation technologies transform how we store, manage and move data.
The data center will drive this disruption, and be shaped by it. Machine-to-machine (M2M) technologies will generate enormous volumes of data, which will be expensive to move. This is what we mean when we say data tonnage – a volume of data that is large enough that we have to think about it differently than the data we created last year.
Data tonnage creates challenges in both the distribution and concentration of data. As datasets grow larger, they are testing the network requirements for analytics, AI and other data-intensive applications.
The answer is two-fold: The first piece is Bigger and faster networks. The second piece distributed compute capacity to perform “data thinning” before sending business-critical datasets across the network. This is edge computing, which is the hot buzzword these days.
But here’s why: A couple months back, analyst Kelly Morgan from 451 Research published a study that looked at the difficulty of moving large datasets. She looked at the time and cost needed to move a volume oif data over a 1000 gigibit per second network connection. For 1 terabyte of data, it was 88 seconds and about $150. For a petabyte of data, it takes a full day, and costs about $30,000 dollars.
That reminds us of the old saying by computer scientist Andrew Tanenbaum – “Never underestimate the bandwidth of a station wagon full of tapes hurtling down the highway.” There’s lots of video footage that is still loaded onto trucks and planes.
This is having a growing impact on the geography of the data center industry. Data gravity will create larger and larger concentrations of compute and storage resources at the core of the network – which will mean business growth at major data center hubs.
Enterprises will tackle data gravity by bringing their applications closer to data sources. It also creates the potential for new extensions of cloud campuses, like CyrusOne’s plan to build a multi-tenant project near a Google data center cluster in Council Bluffs, Iowa.
The AI Arms Race Alters the Compute Equation
Artificial intelligence (AI) plays a starring role in this data tsunami. AI is a hardware-intensive computing technology that will analyze data both near and far. That includes everything from algorithm training at cloud campuses to inference engines running on smartphones.
AI can make products and services smarter. Every business yearns for that, which is why AI is emerging as a strategic priority.
The venture capitalist Fred Wilson summed this up by saying that “Machine learning is now table stakes for every tech company, large and small. Using sophisticated machine learning models to personalize and improve your product is not a nice to have. It is a must have.”
That’s driving a hardware arms race, featuring more innovation than the chip sector has seen in years. Intel says AI is creating an “insatiable “demand for faster, more power-efficient computing hardware.
It will be a busy year for Intel, and fellow incumbents NVIDIA and AMD. in 2020 they’ll be joined by a cluster of AI hardware startups bringing their products to market. An early example is Cerebras Systems, which just debuted a system packing 400,000 compute cores into a 15U rackmount chassis.
These are some eye-popping specs, and as you might expect, they have implications for the data center, including much higher rack densities and more liquid cooling.
The first Cerebras system will use 20 kW of power in a 15U form factor, which implies a rack density of 60kW. That’s why it is liquid-cooled, and we can expect to see more liquid-to-the-chip and immersion solutions be deployed to cool this new AI gear.
This week I saw an example of how these first two trends intersect. It’s an example from the world of AI involving a machine learning model known as GPT-2, which is effectively an AI-powered text generating system that can create synthetic content that looks like it was written by a human. This model is controversial because of concerns that it could be used for generating deceptive content – so-called “deepfakes.”
The other thing that’s notable about GPT-2 is that it’s huge and really resource-intensive. The model evaluates 1.5 billion parameters. And it’s not alone. New machine learning models from Salesforce, NVIDIA and Google are even larger and more compute-intensive.
So it got my attention last week when I came across an article on a data science blog, and it was titled: “Too Big To Deploy: How GPT-2 is Breaking Servers.” The article discussed how these models are getting so large that they are becoming challenging to deploy in production, and embedding them in applications is difficult. They are measure in gigabytes and not megabytes, and are hungry for compute and memory.
The article was on a blog called Towards Data Science, and I’ll include the link in our show notes. One potential solution is to deploy these AI models on cloud platforms, since they can’t run on most user computers or phones. But that could easily lead to unpredictable resource loads and large cloud bills.
The other solution is more horsepower. CPUs alone won’t solve this challenge. AI workloads require GPUs, and other specialized chips like FPGAs and ASICs and Google’s custom liquid-cooled Tensor Processing Units.
This might seem like an outlier today. But keep in mind that the extreme use cases from two years ago, or even a year ago, are commonplace today.
The data tonnage challenge will be real, as will the AI arms race. These are just two of the trends that will shape the data center sector in 2020. There’s lots more ahead in coming weeks, so I want to ask a favor – please take a moment to subscribe to our podcast on Apple Podcasts or wherever you get your podcasts. If you find it useful, tell your friends and colleagues about the Data Center Frontier Show. Where we tell the story of the data center industry … one podcast at a time. Thanks for listening!
About the Author
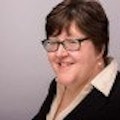