PRINEVILLE, Ore. – In a row of racks deep inside a massive data center sits the engine of Facebook’s ambitions in artificial intelligence. Each server chassis is packed with computing horsepower, including graphics processing units (GPUs) that can crunch enormous volumes of data.
This is Big Sur, and it is the key to Facebook’s bid to create a smarter newsfeed for its 1.6 billion users around the globe. Using Big Sur, Facebook can train its machine learning systems to recognize speech, understand the content of video and images, and translate content from one language to another.
Machine learning holds tremendous promise, but requires a lot of horsepower. The gains in performance and latency provided by Big Sur help Facebook crunch more data, dramatically shortening the time needed to train its neural networks.
Facebook has deployed thousands of Big Sur servers in its data centers, cireating a platform with 40 petaflops of computing power, which would rank it among the world’s most powerful systems.
DIY Approach to Machine Learning
Each Big Sur unit consists of a 4U chassis, which houses eight NVIDIA M40 GPUs and two CPUs, along with SSD storage and hot-swappable fans at the rear of the server.
Neural networks mimic how the human brain works, allowing computers to adapt and “learn” tasks without being explicitly programmed for them. As leading tech companies push the boundaries of machine learning, they are often following a do-it-yourself approach.
Big Sur is a 4U chassis packed with eight NVIDIA M40 GPUs . The bright green ccover protects the motherboard and CPUs, but also assists in cooling. (Photo: Facebook)
“There wasn’t any good hardware on the market at the time, so we developed our own,” said Kevin Lee, a Technical Program Manager for Facebook. They reached out to NVIDIA, whose graphcs processing units were initially focused on accelerating video games, but now have seen broad adoption in high-performance computing.
“We work very closely with Facebook Research,” said Ian Buck, Vice President of Accelerated Computing at NVIDIA.
Getting Parallel, Getting Results
Using CPUs, it took three months to train a machine learning neural network. Adding GPUs reduced that processing time to a month, Buck said.
Facebook and NVIDIA began working together to optimize a design using the NVIDIA Tesla M40 GPU accelerator, the company’s flagship product for deep learning.
Each M40 GPU houses 3,072 NVIDA CUDA computing cores, small processors that work together on computing tasks. By working in parallel with many processors at once, GPUs can accelerate many types or workloads.
“With Big Sur, you can now train a neural network in less than a day,” said Buck.
Facebook has optimized these new servers for thermal and power efficiency, allowing them to operate alongside standard CPU-powered servers in Facebook’s data centers. In Prineville, the Big Sur cluster shares space with Open Compute servers inside PRN2, a 350,000 square foot server farm that is longer than an aircraft carrier.
A view of Big Sur with the cover removed, exposing the motherboard, CPUs and DIMM memory. (Photo: RIch Miller)
Each Tesla GPU can use up to 300 watts of electricity, bringing the power footprint for each Big Sur chassis to about 2.5 kilowatts. Facebook likes to keep power densities below 12 kW a rack, so it houses four Big Sur units in each rack, resulting in a power footprint of 10kW per rack.
Like most Open Compute designs, Big Sur’s components are arranged to optimze airflow through the chassis. Each Big Sur unit features a bright green plastic over over the motherboard and processors. This protects the components, but is also designed to enhance the airflow, narrowing near the back to create a “Venturi effect” that accelerates the air movement across the GPUs.
Smarter Services Through AI
Facebook isn’t alone in prioritizing artificial intelligence and machine learning. Google, Apple and Amazon have also created research labs to pursue faster and better AI capabilities. They have used different approaches to hardware, with Google opting for custom ASICs (application specific integrated circuits) for its machine learning operations.
Whatever the hardware approach, the goal is the same: take elements of digital assistants like Apple’s Siri and the Amazon Echo and build them into almost every element of their technology and services.
Facebook’s Lee said the company is already using Big Sur to train neural networks to “read” to the blind, recognizing images and sharing a spoke description of their contents. Buck and Lee demonstrated how Facebook;s AI can review thousands of paintings, recognize desired visual elements in the painting, and incorporate them into a new work of art
In the same way, Facebook’s servers and algorithms can learn from the content shared by its users, and tailor the updates it selects for each person’s newsfeed. Artifical intelligence aligns with Facebook’s culture of rapid iteration, constantly testing new approaches to improving its products.
“The whole point is to go fast,” said Ken Patchett, Facebook’s Director of Data Center Operations at Prineville. “We have to get better quickly.”
About the Author
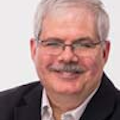