Inside NVIDIA’s Vision for AI Factories: Wade Vinson’s Data Center World 2025 Keynote
As artificial intelligence accelerates the reshaping of the digital infrastructure landscape, Nvidia has emerged as a central force not just in silicon, but in reimagining the data center itself. At Data Center World 2025, Wade Vinson, Nvidia’s Chief Data Center Distinguished Engineer, offered a rare and detailed look under the hood of this transformation, laying out the company’s vision of the AI factory—a new archetype for hyperscale infrastructure engineered for a trillion-token era.
“Every single data center in the future is going to be power-limited,” Vinson told a packed hall in Washington, D.C. “And your revenue is limited if your power is limited.” It’s a mantra that echoes from the highest echelons of Nvidia strategy, first voiced by CEO Jensen Huang at GTC and now embedded in the design ethos of what Vinson calls “AI factories”—facilities capable of supporting 100,000 GPUs or more, operating at gigawatt scales, and optimized to convert grid power into model tokens with ruthless efficiency.
In a moment that underscored both emotional connection and professional pride, Vinson introduced drone footage of a live 1-gigawatt AI factory currently under construction—an operational blueprint of the massive-scale builds Nvidia is driving in collaboration with partners like Crusoe. “Right in the middle of the picture you see these two little gray boxes,” he said. “That is the substation to convert the 1.21 gigawatts—yes, gigawatts—of electricity that this factory needs.”
Each visible “four-pack” in the video, Vinson noted, represents a 25-megawatt hall, bracketed by advanced liquid and air cooling infrastructure, with onsite generation powered by ten natural gas turbines. “What’s kind of cool,” he added, “is that this is not so different from the smallest data centers. A 25-megawatt hall is still rows of hot aisle containment and distribution—so if you're in this room, you're already on the journey.”
Vinson’s keynote didn’t just chart a vision of hyperscale ambition—it rooted that vision in pragmatic industry transition. With a nod to Nvidia’s internal metric—“grid-to-token conversion efficiency”—Vinson emphasized that any watt not directly contributing to inference or training is effectively lost revenue for AI customers. “It’s not as sexy as PUE, I admit,” he joked, “but maybe time will tell.”
At the heart of Nvidia’s architectural innovation is the integration of the company's Omniverse Blueprint and digital twin technology. Vinson highlighted how Nvidia’s engineering teams now use high-fidelity simulation tools—leveraging CUDA, Cadence Reality, and Schneider Electric’s ETAP platform—to co-design power and cooling systems in parallel. “We can run what-if scenarios in seconds instead of hours,” he said, describing how digital twins reduce construction errors, accelerate retrofit timelines, and optimize total cost of ownership.
From gigawatt builds to 3–5 MW retrofits in repurposed retail spaces, Vinson argued that the AI factory era doesn’t necessarily require new greenfield development. “That’s the old Compaq manufacturing plant in Houston,” he said, pointing to a converted 20 MW facility. “And that’s a mall in San Francisco—look at all that rooftop photovoltaic potential.”
Whether it’s mills in the Northeast powered by rivers or colo space in Equinix San Jose, Vinson’s point was clear: the future is already here—it’s just not evenly distributed.
AI Factories at Every Scale—and a $100 Trillion Opportunity
Vinson next turned to the GB200-powered systems—Nvidia’s flagship Grace Blackwell platform—to illustrate the continuum of AI factory deployments, from hyperscale to edge-scale. “This is one of my favorite pictures,” he said, gesturing to a slide of a 120-kilowatt deployment. “I’ll call it the smallest instance of a true AI factory. And look at all the vendors building it.”
Despite its compact footprint, Vinson emphasized that the system embodied the same architectural principles Nvidia applies at gigawatt scale: dense compute, efficient power conversion, workload optimization, and scalable orchestration. “This isn’t about onesie-twosie deployments,” he noted. “This is an industrial model. It’s replicable, and it’s being built at volume.”
The reason for that scale, Vinson argued, is macroeconomic. “Today, the world economy—across computing, healthcare, transportation, manufacturing—AI is touching every single sector,” he said. Global GDP, he noted, stands above $100 trillion. “If we get even a 10% boost in productivity from AI over the next 15 years, that’s a $100 trillion value creation. That’s more than the electrification of the planet.”
It’s a sweeping claim, but one backed by compute curves. Vinson delved into the shifting contours of AI workloads, particularly the explosive growth in post-training and reasoning. “Most people understand pre-training—a lot of compute to generate intelligence. But what’s changed is the post-training step. You have to send the model to school.”
Rather than hand-coding subject matter understanding—what a cat is, a protein, or a gas molecule—organizations want to automate domain adaptation. And that requires serious horsepower. “Post-training can take 30 times more compute than pre-training,” Vinson said. “More compute means more data centers.”
He continued with another example: inference via agent-based reasoning. “Ask ChatGPT a question, you get one answer. Ask an agent, and it launches 50 models, compares their answers, and picks the best one. That’s called reasoning. It takes 100 times the compute.”
The implication is clear: As capabilities scale, infrastructure must too. “As you move along the X-axis—more compute—you’re going to need more and more data centers,” Vinson said, describing what he calls the “AI Factory Curve.”
The New Metrics of AI Infrastructure: From Blackwell to Dynamo
Vinson then introduced the conceptual framework Nvidia uses to evaluate AI factory performance: maximizing transactions per second per watt (Y-axis), while delivering fast, satisfying results for users (X-axis). “It’s not just about peak performance. It’s about what the customer sees—their affinity to your AI factory,” he explained.
Each dot in Vinson’s performance graph represented a different model or workload across multiple industries. From legacy Hopper platforms to Grace Blackwell to the NVL72 superchip architecture, the trend was unmistakable: greater efficiency, tighter orchestration, and lower latency. “With NVL72, we created a system that looks like one giant GPU,” Vinson said. “And now we have Dynamo.”
Dynamo is Nvidia’s newly unveiled AI factory operating system, purpose-built to optimize the entire cluster stack—from job scheduling to workload prioritization. “It’s smart enough to reprioritize resources on the fly,” Vinson said. “We didn’t get more throughput on the Y-axis, but look at how much faster it answered the question.”
Faster responses, he emphasized, translate to customer stickiness and long-term competitive differentiation. “That’s what your customers want. That’s what drives them to your factory instead of someone else’s.”
Vinson closed the segment with a dense but revealing “eye chart” slide showing workload mapping across Nvidia’s AI stack. “I love it,” he said, “because it explains just how much time and effort we’re putting into this.”
Designing the AI Factory: From Blackwell to Kyber and Beyond
To truly understand the scale and ambition of NVIDIA’s AI factory vision, Vinson invited attendees to imagine something familiar — a Honda factory. “One part might be building motorcycles, another race cars,” he said. “My Civic hatchback isn’t coming off the same line as your Acura RS.”
The analogy speaks volumes: each AI workload has unique demands, but the factory must flexibly support them all — from inference to reasoning, from 1,000-token prompts to massive 32,000-token input models — and deliver consistent high-throughput, low-latency performance across the board.
These workloads are only getting larger. Vinson cited a 40x performance improvement using a single rack for the larger model set, underscoring how tightly integrated systems design is becoming the ultimate determinant of performance-per-watt.
The Factory Runs on Silicon, Systems—and Strategy
“The revenue your customers earn is going to be dictated not just by the efficiency of the factory design, but the efficiency of what goes inside it,” Vinson said, segueing to Jensen Huang’s famed roadmap slide — now a staple of every major NVIDIA announcement. Blackwell is shipping now, Blackwell Ultra is on deck, and Rubin is in development. Alongside these, the Kyber rack — NVIDIA’s latest architectural leap — reflects the company’s scale-up philosophy in hardware and systems. From Hopper to Blackwell to Kyber, each step represents a generational leap in factory output.
Vinson described the Kyber rack as the physical embodiment of NVIDIA’s modular, hyper-efficient design ethos. “If you look at all the components inside an Oberon rack,” he said, “it works together seamlessly.” The Kyber rack takes this further — and larger. “We’re designing racks that are no longer just rows of GPUs. They’re waves — harmonized orchestration of compute, cooling, and power.”
AI Factory ≠ Traditional Data Center — But Close
So what makes an AI factory fundamentally different from a traditional data center? “It’s the interconnectedness,” Vinson explained. “Everything wants to think together.” Higher power, liquid cooling, smarter scheduling — yes. But also familiarity. “To the engineers in the room: it still looks a lot like the data center you’re building today. It just needs to be smarter, denser, and purpose-built for tokens.”
The future, he said, lies in dry-cooled, waterless systems. NVIDIA is targeting 88% of AI factory cooling to be handled this way by the Rubin generation. A 400kW unit using dry cooling consumes just 2% of system power on the worst day of the year — and under 1% annually. “It’s 25% more power going to tokens,” said Vinson. “That’s a free quarter of a data center you didn’t have before.”
The racks themselves are changing too. Gone are the air-cooled CPU-friendly chassis of Hopper’s era. The Blackwell Ultra era demands direct water connections, high-density power, and OCP-compliant MGX power infrastructure — donated by NVIDIA to the open community. “With 48 individual rectifiers per rack, we get innovation headroom,” said Vinson, predicting another 3–6% gain in usable compute over the next two generations from power architecture alone.
Optics, Uplinks — and the Death of the CPU?
Another drain on power? Lasers. “Lasers are expensive and fussy,” Vinson said. By aggregating links and reducing optical I/O per switch, NVIDIA has shaved 3–5% more power — in a 100,000-GPU data center, that’s no small feat.
Retrofits — or as Vinson rebranded it, “reloads” — are the final frontier. “There are 100 gigawatts of colo space running on 5-to-7-year-old CPUs,” he said. “Why replace them with more CPUs?” He said that with CUDA-X and vertical ISV support, Blackwell is now eating the CPU’s lunch — not just for AI and ML, but for full-stack enterprise apps across oil & gas, healthcare, and financial modeling. “Every software vendor is seeing 20 to 50x speedups,” Vinson said. “This is how you monetize your infrastructure.”
Engineering the Future, Nut by Bolt
Next, Vinson turned to a slide showing his team’s CAD model — every nut, bolt, wire, capacitor — the full digital twin of NVIDIA’s AI factory vision. “This is how we build,” he said. “Because in this era, the real advantage isn’t just silicon — it’s system thinking.”
Building the Future Before It’s Built: How Digital Twins and Real-World Iteration Are Shaping Nvidia’s AI Data Center Strategy
For Vinson, the key to building future-ready AI infrastructure isn’t just advanced hardware — it’s advanced modeling. “[Data Center World Lifetime Achievement award honoree] Christian [Belady, Senior Advisor, DigitalBridge] and I were doing modular data centers 25 years ago,” he recalled. “We modeled everything — because you simply cannot achieve this quality, at this scale, in the field without modeling first.”
The concept of the digital twin — a real-time, data-driven virtual replica of physical infrastructure — has become central to Nvidia’s ability to test and deploy high-density GPU clusters at hyperscale speed. Vinson emphasized that while design software like AutoCAD, NavisWorks, BIM, and SolidWorks are foundational, a true digital twin goes far beyond static blueprints. “If you’ve got a fully instrumented real-life model, the simulation is as good as the real thing,” he said. “We use reinforcement learning, we feed real-time data back into the model, and the system gets smarter.”
This iterative modeling loop, powered by live telemetry and AI-driven optimization, has enabled Nvidia to push the limits of power and thermal design with confidence. For example, the company’s 2.5MW hot aisle containment (HAC) module — featuring 1,152 GPUs — was a generational leap over the earlier 504-GPU, 1MW Hopper deployment. Achieving that leap required not just design innovation, but validation against thousands of permutations. “We remodeled and iterated 35,000 designs,” said Vinson.
The result was a modular, water-assisted HAC design that could be deployed in a colo parking lot or on a campus rooftop, borrowing cooling water or power as needed — and still maintaining operational integrity. That kind of rapid experimentation wouldn’t be feasible without digital twins.
Looking ahead, Nvidia is aiming for even more efficient scaling, landing on 5 megawatts as the “sweet spot” for future deployment modules — whether for a university, enterprise, or regional AI hub in places like Abilene or Memphis. This size, Vinson explained, is not only scalable, but future-proofed. “If we design it for 5 megawatts today, your customers will be ready for two generations of GPUs ahead,” he said, referencing Nvidia’s roadmap from Blackwell to Ultra, then Rubin and Feynman.
In fact, Nvidia’s approach treats power and density as variables to be continuously optimized. “If that power goes up? Take the power from two racks and run it into one,” Vinson proposed. The physical infrastructure surrounding the rack — UPS systems, switchgear, cooling loops — stays fixed, while rack power density climbs. “That’s the Jensen comment,” he noted, referring to Nvidia CEO Jensen Huang’s observation that every data center is power-limited at its perimeter.
In practice, this means reducing from 24 racks in an early HAC design to as few as three high-power racks in the future — without expanding the data center’s footprint. Nvidia is already testing power sidecars that slot between racks and site power to manage these increasingly intense loads.
And these aren’t just theoretical models. “The digital twin is worthless if you didn’t build a real one,” Vinson stated bluntly. To prove it, Nvidia recently partnered with Equinix to retrofit a real-world facility — originally designed as a traditional 5kW air-cooled cage — with Blackwell GPU racks, power delivery upgrades, and water-cooled dry coolers. The results mirrored the digital model with uncanny accuracy. “In real life, it almost looked easier,” said Vinson. “You could see that we could double the network cabling, move power across racks, and still fit everything inside the existing envelope.”
Even the lessons learned during install were fed back into the model in real time. “Somebody says, ‘It doesn’t fit.’ Well, you’ve got a digital twin, how can it not fit?” Vinson laughed. “Turns out, the as-built didn’t match the model. So we fixed the model.”
From cabling and power delivery to leak detection and control valving for warm water loops, Nvidia’s reference designs are evolving through a tightly coupled feedback loop of simulation and field implementation. “If we proved it in a 15-year-old air-cooled data center,” Vinson concluded, “we can prove it anywhere.”
From 'Prettier Water' to 'Every Watt Matters': The Practical Art of AI Factory Deployment
For all the engineering precision and GPU muscle Nvidia is bringing to bear on AI infrastructure, Wade Vinson ended his talk on a deeply practical note: make it work — then make it beautiful. He gestured toward the piping, power panels, and valving that enabled the retrofit of a legacy 2.5MW air-cooled data hall into a next-gen AI pod. “We’re going to make prettier water,” he joked. “But I’m a mechanical thermal engineer — we made it work. That’s the prettiest thing of all.”
Vinson wrapped with a real-world view inside what he calls “the cave” — the retrofitted data center space now housing a full-scale Blackwell deployment. “People think I’m exaggerating when I tell this story,” he said, “but there was a room that had two and a half megawatts of air-cooled kit. Now? It’s just got one superpod.” He pointed to a 250-amp row power panel — one of 24 needed to feed the new dense racks — and emphasized that re-powering old infrastructure is not only possible, but imperative.
So what’s the call to action? Vinson was direct: don’t wait. If you have a lot — an old colo site, a dormant manufacturing facility — you’re sitting on opportunity. “Don’t wait for an interconnection study,” he urged. “We’re showing you these tools — the digital twin lets you design and validate today. Get to token creation now.”
Every watt, he stressed, must be maximized across the full runtime of the year — 8,766 hours. Nvidia’s own modeling showed that a 16MW deployment, run efficiently enough, could potentially yield 28MW worth of AI output annually — an idea that makes traditional data center operators nervous. “I think I scared some friends at Digital Realty,” Vinson smiled. “They said, ‘Sure, Wade, just cut a check and we’ll buy new gear from Schneider.’”
But for Vinson, this is no longer about hardware arms races. “As Jensen said, it’s no longer ‘the more you buy, the more you save.’ Now? It’s the more you make.”
And making — in this new era of AI factories — requires not just bravery and power, but world-class modeling and engineering discipline. “It’s not just about the guts,” he told the audience. “You need really great models. And we’re here to help you do that.”
In closing, Vinson left the room with a new mantra — one that may soon find its way onto the walls of data centers across the world:
#EveryWattMatters
At Data Center Frontier, we talk the industry talk and walk the industry walk. In that spirit, DCF Staff members may occasionally use AI tools to assist with content. Elements of this article were created with help from OpenAI's GPT4.
Keep pace with the fast-moving world of data centers and cloud computing by connecting with Data Center Frontier on LinkedIn, following us on X/Twitter and Facebook, as well as on BlueSky, and signing up for our weekly newsletters using the form below.
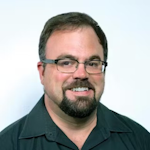
Matt Vincent
A B2B technology journalist and editor with more than two decades of experience, Matt Vincent is Editor in Chief of Data Center Frontier.